How Long Does It Take to Learn Machine Learning?
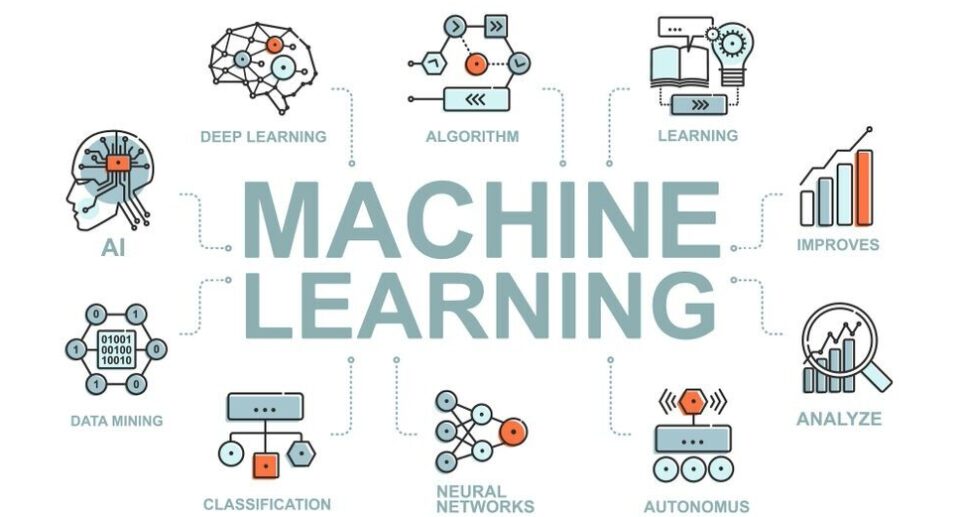
In an era marked by rapid technological advancement, machine learning (ML) stands out as a transformative force across various industries, from healthcare to finance. Its ability to improve efficiencies, enhance predictive accuracy, and unlock new capabilities has made it a critical skill in the tech-driven job market. As more individuals consider pivoting or advancing their careers in this dynamic field, one of the most pressing questions is: How long does it take to learn machine learning? Understanding the time commitment required can help you plan your learning path more effectively, whether you’re starting from scratch or looking to deepen existing expertise.
Understanding Machine Learning
Machine learning (ML) is a subset of artificial intelligence (AI) that focuses on the development of systems that can learn from and make decisions based on data. Unlike traditional software models that require explicit programming for every step, machine learning uses algorithms that can analyze data, learn from it, and make predictions or decisions without human intervention.
Key Concepts of Machine Learning
- Supervised Learning: This involves training an algorithm on a labeled dataset, meaning the data includes an answer key that the model can learn from. For example, a supervised learning algorithm could be trained to recognize emails as either spam or not spam by learning from a set of emails pre-labeled by users.
- Unsupervised Learning: In contrast, unsupervised learning algorithms are used when the data has no labels. Here, the system tries to learn the underlying patterns and structure from the data itself. An example could be identifying customer segments in marketing data based on purchasing behavior.
- Reinforcement Learning: This type of learning uses a system of rewards and penalties to compel the computer to solve a problem by itself. Gaming, navigation, and real-time decision-making are common areas where reinforcement learning is applied.
Applications of Machine Learning
The applications of machine learning are vast and touch virtually every industry today, making its study and understanding increasingly important. Here are a few examples:
- Healthcare: ML models can predict patient diagnoses based on past medical records and current symptoms, improving treatment accuracy and patient outcomes.
- Finance: Algorithms can analyze market conditions, client portfolios, and economic trends to provide financial advice or automate trading.
- Retail: Machine learning enhances customer experiences through personalized shopping recommendations based on past purchase history and browsing habits.
- Transportation: Self-driving cars use machine learning to make real-time navigation decisions based on sensor data.
Impact on Daily Life
Machine learning’s integration into daily life continues to deepen, evidenced by personal assistants like Siri and Alexa, which use ML to interpret and respond to voice commands. Recommendation systems in platforms like Netflix and YouTube personalize user experiences by suggesting shows and videos based on past viewing behavior. These technologies are not just conveniences; they represent significant advances in how machines interact with human behaviors and preferences.
Factors Influencing Learning Duration
The journey to mastering machine learning varies significantly among learners, influenced by factors such as:
- Prior Experience: Background in programming and statistics can dramatically reduce the learning curve.
- Concept Complexity: Fundamental concepts may require weeks to comprehend, while advanced techniques could take years to master.
- Commitment: The extent of your time commitment, whether as a hobbyist or a professional pursuing a career change, impacts how quickly you progress.
Types of Machine Learning Courses
Machine learning education is accessible through various platforms, offering flexibility to fit different learning preferences:
- Online Courses: Ideal for those balancing other commitments, online courses allow for self-paced study. Platforms like SimpleAcademy offer comprehensive machine learning online courses that cater to beginners and advanced learners alike.
- In-Person Bootcamps: These are intensive and immersive, suited for individuals seeking structured and fast-paced learning environments.
- University Programs: For deep dives into theoretical aspects, university-led courses provide extensive knowledge but require a significant time investment.
Expected Timeline for Different Learning Stages
- Basics: Beginners can grasp foundational concepts within a few months through online courses or bootcamps.
- Intermediate Proficiency: Achieving a working knowledge of machine learning algorithms and tools might take an additional 6-12 months of study and practical application.
- Advanced Expertise: Mastering complex topics and becoming industry-ready could take several years of dedicated learning, especially if pursued part-time.
Key Resources and Platforms
For those embarking on their machine learning journey, choosing the right educational resources is crucial for building a strong foundation and advancing through to mastery. The best online courses in machine learning provide not just an introduction to basic concepts but also a structured pathway that guides learners from novice to expert levels. Here’s what to look for in top-tier machine learning courses:
Comprehensive Curriculum
- Foundational Learning: Look for courses that start with the basics of Python programming, statistics, and data analysis, essential for those new to the field.
- Intermediate Exploration: As you progress, the curriculum should cover more complex algorithms and machine learning models, including supervised and unsupervised learning techniques.
- Advanced Studies: The most comprehensive courses will advance to deep learning and artificial neural networks, preparing you for specialized fields like natural language processing or computer vision.
Flexible Learning Options
- Self-Paced Learning: Many platforms offer courses that allow you to learn at your own speed, accommodating your schedule and pace of learning.
- Mentorship and Support: Some programs enhance learning experiences by providing access to mentorship from experts, which can be crucial for overcoming challenges and gaining deeper insights.
- Hands-On Projects: Courses should include practical projects that allow you to apply what you’ve learned in real-world scenarios, solidifying your knowledge and building your portfolio.
Community and Networking
- Collaborative Learning Environments: Engaging with a community of fellow learners can greatly enhance your learning experience. Look for courses that offer forums, group discussions, and peer networking opportunities.
- Industry Connections: Some educational platforms also provide networking opportunities with industry professionals, which can be invaluable for career advancement.
Accessibility and Support
- Resource Availability: Ideal courses offer extensive resources such as interactive tutorials, video lectures, and continuous assessments to ensure you can review and understand the material fully.
- Customer Support: Reliable customer support can help navigate any technical or administrative issues, making your learning process smoother.
By choosing a resource like SimpleAcademy.ai, which is known for its robust course offerings that cater to all levels of proficiency, learners can be assured of a high-quality education that prepares them for real-world applications of machine learning. Whether you’re just starting out or looking to deepen existing knowledge, SimpleAcademy.ai provides the tools and community support needed to succeed in this dynamic field.
Strategies to Accelerate Learning
Effective learning strategies include:
- Hands-On Practice: Engage with real-world datasets and projects.
- Community Learning: Join forums and groups to exchange knowledge and tackle challenges collaboratively.
- Consistent Practice: Regular review and application of concepts help solidify understanding.
Conclusion
The journey to mastering machine learning is as varied as the learners who embark on it, encompassing a range of personal, professional, and educational factors that influence the pace of learning. While it can be challenging to pinpoint an exact timeframe for becoming proficient in ML, a committed and structured approach can significantly enhance the learning experience. By leveraging key resources, engaging actively with the community, and maintaining a consistent practice regimen, you can accelerate your mastery of machine learning. Remember, each step in this journey not only increases your technical skills but also your ability to innovate and solve complex problems in real-world scenarios. Embrace the learning process, and let your curiosity and dedication guide you towards becoming an adept machine learning professional.